Final December, Scott Gavura wrote a good piece at SBM, presenting the work of a brief paper revealed in JAMA Inside Medication wherein the authors introduced how AI and Massive Language Fashions (LLMs) like ChatGPT might be manipulated to supercharge well being misinformation and unfold faux information in social media, calling for the necessity of regulatory frameworks and human monitoring by well being professionals.
Though the specter of utilizing Massive Language Fashions (LLMs) for misinformation, disinformation, and malinformation (MDM) in science is certainly actual, the proposed options involving regulatory frameworks and human-based countermeasures appear subpar in comparison with the extent of risk we face.
Preventing fireplace with fireplace
If we have now realized something from the Age of the Web, it’s that cyber threats have been most successfully challenged and countered utilizing the identical cyber instruments for protection.
Regardless of the introduction of quite a few regulatory frameworks and authorized instruments over the a long time towards cyber-deception, our digital historical past is replete with success tales the place an attacking device was repurposed as a defensive one, together with the hacker wars of the ‘90s, the place authorities companies employed ex-hackers to defend their methods or determine weaknesses, to the ingenious protection mechanism invented to guard e-mail inboxes from spam: the Junk Folder.
It’s solely logical
Equally, within the context of AI-driven MDM assaults on science, using LLMs and associated AI fashions as instruments towards MDM is logical. I imply, if these instruments are much more environment friendly at producing faux data than people, then solely AI instruments can assist us defend successfully towards it, proper?
That is finest articulated in a research from the Pennsylvania State College by Lucas et al (2023), the place they remark:
“To fight this rising danger of LLMs, we suggest a novel “Preventing Hearth with Hearth” (F3) technique that harnesses fashionable LLMs’ generative and emergent reasoning capabilities to counter human-written and LLM-generated disinformation.”
That’s spot on, though the authors’ suggestion shouldn’t be precisely novel. Greater than a 12 months in the past, when LLMs began getting extra publicity, Edward Tian, a 22-year-old scholar at Princeton College immersed in finding out GPT-3 — which is the earlier model of ChatGPT — developed an app to determine content material created by AI, in simply three days. His app employed the machine studying mannequin of ChatGPT in reverse, inspecting texts for indicators attribute of AI-produced materials.
Nonetheless, a small early research on Tian’s device reported that the app had a low false-positive (classifying a human-written textual content as machine-generated) and a excessive false-negative price (classifying a machine-generated textual content as human-written). Nobody anticipated it could be simple. However it was a begin.
Countering LLM-generated Faux Information
Quite a few research have examined the ‘F3 technique’ of using LLMs to counteract LLM-generated faux information. Anamaria Todor and Marcel Castro, Principal and Senior Options Analysts at Amazon Internet Providers, respectively, have investigated the potential of LLMs in detecting faux information. They argue that LLMs and AI-related instruments are adequately superior for this process, particularly when using particular immediate strategies just like the Chain-of-Thought and ReAct patterns, together with data retrieval instruments.
Searching for patterns
For these much less acquainted with ChatGPT and related LLMs, ‘patterns’ consult with the assorted methods a consumer formulates instructions or directions to the LLM, aiming to enhance the probability of acquiring a desired outcome. Abusers typically exploit these patterns to craft misinformation that’s as convincing as potential. Todor and Castro developed a LangChain utility which, upon receiving a information merchandise, can analyze its language sample to find out whether or not the article is genuine or fabricated.
And that is very fascinating. A variety of research have confirmed that language performs a vital function in detecting AI-driven MDM. Plainly the best way language is utilized by these AI fashions has some distinct variations from the best way people use it, which might be exploited and assist us determine it. Imane Khaouja, an NLP Researcher and Information Scientist who examined an AI mannequin known as CT-BERT, wrote in a current weblog submit about the best way that these AI instruments can use language as a marker for detecting misinformation:
“Upon analyzing (..), it turns into obvious that actual tweets have a tendency to make use of scientific and factual language. (..) Actual tweets are characterised by a reliance on goal data, logical reasoning, and a dedication to accuracy. In distinction (..) faux tweets predominantly depend on emotional and sensationalist language. These articles typically include exaggerated claims, lack factual proof, and make use of emotional and fearmongering techniques to govern readers’ feelings. The usage of such language and strategies goals to impress sturdy emotional responses and seize the eye of the viewers, doubtlessly resulting in elevated engagement and sharing of the content material.”
“COVID-Twitter-BERT”
CT-BERT, which stands for “COVID-Twitter-BERT”, is an AI-model developed by a Swiss-Spanish search collaboration. The mannequin is pre-trained on a big corpus of COVID-19 associated Twitter posts and might be utilized for varied pure language processing duties corresponding to classification, question-answering, and chatbots.
The authors didn’t design CT-BERT to battle misinformation on COVID-19, however a Russian analysis staff used CT-BERT to win the “COVID-19 Faux Information Detection Problem” on the 2021 convention of the Affiliation for the Development of Synthetic Intelligence (AAA 2021). In that competitors, 166 analysis groups from world wide used their AI fashions towards a set of twitter posts that introduced misinformation about COVID-19, and their efficiency was scored. The CT-BERT mannequin of the profitable staff achieved a weighted F1-score of 98.69 on the check set, profitable the primary place in that competitors.
Human-written disinformation vs LLM-generated variants
Going again to the research by Lucas et al., their findings relating to the function of language had been significantly intriguing. First, they noticed that ‘LLMs wrestle extra to detect human-written disinformation in comparison with LLM-generated variants.’ This consequence is considerably anticipated, contemplating the variations in language utilization by the 2 creators, people and LLMs.
Second, they famous that LLMs had been more proficient at figuring out misinformation in prolonged information articles than briefly social media posts. This may be attributed to the restricted language briefly articles, making them more difficult to categorise efficiently. Nonetheless, Lucas et al. reported promising ends in their mannequin’s capacity to detect misinformation inside an enormous array of on-line content material. They concluded that ‘assessing detection and disinformation mitigation can be vital as LLMs proceed to advance.
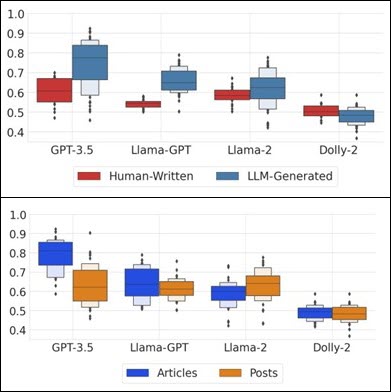
Accuracy (F1) scores on detecting LLM-generated disinformation and human-authored disinformation (above) and on evaluating the efficacy of various LLMs detecting quick social posts (beneath). Taken from Lucas et al.
The necessity for a extra strong and synchronized technique
Regardless of numerous research, much like Lucas et al, that look at alternative ways we are able to use AI-models and LLMs towards faux data, particularly within the context of science and well being science, the sector continues to be in its infancy. A extra strong and synchronized technique must be applied utilizing varied methodologies.
In a current (unpublished) work by Canyu Chen and Kai Shu from the Illinois Institute of Know-how, the authors reviewed circumstances and research the place LLMs and associated AI instruments can and have been used towards misinformation. They categorize them in three predominant methods:
- Misinformation Detection – utilizing algorithmic-based AI instruments to detect human-produced LLM-produced misinformation (instance),
- Misinformation Intervention – making the most of LLMs’ fast-generating capability to supply stable counter-arguments and reasoning towards introduced MDM, to enhance the convincingness and persuasive energy of the debunking responses (instance) and
- Misinformation Attribution – utilizing AI instruments to determine the creator(s) of MDMs, combating misinformation by tracing the origin of propaganda or conspiracy theories and maintain the publishers or supply accountable.
Timing is the whole lot
One large limitation of utilizing LLMs towards MDM assaults is the timing constraints present in checking factual data. The data contained in most LLMs shouldn’t be up-to-date (i.e. the data cutoff date for GPT-4 is April 2023) so it’s tough to make use of LLMs to test data for info in dates after the mannequin’s cutoff date.
To bypass this hurdle, we have to introduce exterior assist, the place people or machines can assist LLMs by introducing newer knowledge of their studying previous to fact-checking. For instance, Cheung & Lam mixed the retrieved data from a search engine and the reasoning capacity of an LLM to foretell the veracity of varied claims (unpublished). In one other research, Chern et al. proposed a fact-checking framework built-in with a number of instruments, corresponding to Google Search and Google Scholar) to detect the factual errors of texts generated by LLMs (unpublished).
All palms on deck
On this early stage of LLM growth, one factor that’s universally clear from the associated literature in terms of fight MDM utilizing LLMs and associated AI instruments, is that there’s nonetheless a protracted solution to go, however it appears the correct solution to go. Creating MDM continues to be simpler than combating MDM, utilizing the identical instruments. Which is kinda pure, when you take into account that LLMs and ChatGPT entered our lives solely a couple of 12 months in the past or so.
However, utilizing AI-models to detect and publicize deceptors may not be sufficient. All palms must be on deck. Which means utilizing each human device at our disposal to battle MDMs — from inserting regulatory frameworks on LLM growth and use, to forcing builders to incorporate fail-safe mechanisms towards misuse into their fashions.
Three-stage technique
Canyu Chen and Kai Shu mentioned this of their current work, introduced on the thirty seventh Convention on Neural Data Processing Programs (NeurIPS 2023). The authors proposed a three-stage technique for tackling LLM-produce misinformation:
- On the coaching stage, by eradicating nonfactual content material from the LLMs coaching knowledge,
- On the utilization stage, by introducing security valves corresponding to immediate filtering towards utilizing LLMs to supply misinformation, and
- On the affect stage, through the use of LLMs for misinformation detection, intervention and attribution, as described above.
This synchronized and systematic motion may very well be a extra environment friendly and optimum technique to battle AI-driven MDM.
People and AI united
The battle towards AI-driven science and well being misinformation calls for a strategic mix of AI instruments and rigorous regulatory measures. Whereas leveraging LLMs and associated AI instruments for detection and intervention is promising, it’s nonetheless crucial to couple these applied sciences with moral tips and human experience. The trail ahead is difficult however it gives a novel alternative for synergistic options, uniting AI’s computational prowess with human judgment to safeguard scientific discourse. This twin method is essential in navigating the complexities of misinformation within the quickly evolving AI panorama.